Drug Discovery
goals
A US based biotech company aims to increase the quality of life for patients with degenerative diseases by treating and extending their healthspan. The research scientists needed to identify proteins that have rejuvenating properties for treatment of a specific disease called Sarcopenia, a special form of Myopathy, where individuals lose their ability to regenerate their skeletal muscles.
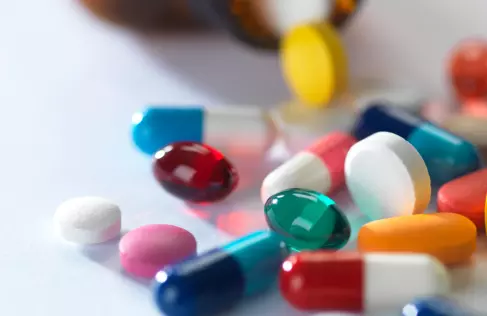
challenges
Research and experimentation was not yielding the number of
proteins needed for the next stage of discovery. Each of the
20,000 proteins had 20,000 features which needed to be
analyzed to identify a true positive.
The traditional approach to assessing each protein during the
search phase woudl cost roughly around
$2,000 per protein, which equated to a cost of
$40 million of all the proteins were evaluated through
wet lab experiments. The client’s goal was to reduce the cost
and tim eof analyzing proteins during the research phase.
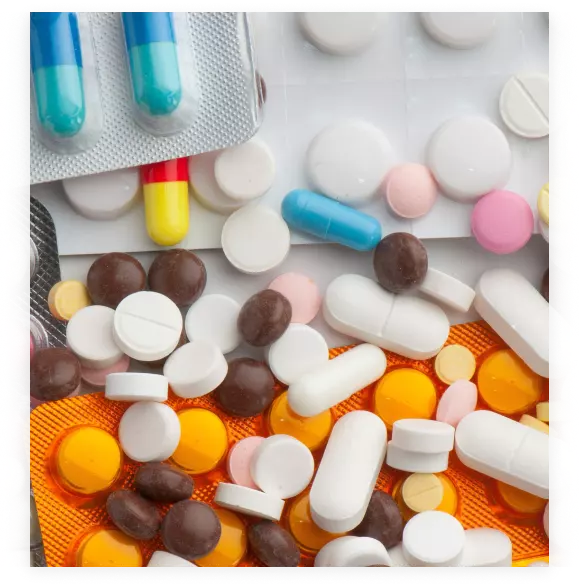
solution
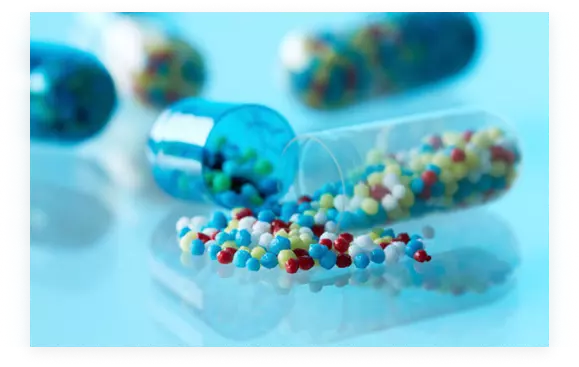
The client wanted to use
NLP to comb through scientific journals and solidify the
protein search based on the specific disease being analysed and
different predicted proteins. This would help speed up the process
by proving the alignment of contemporary scientific literature
with the findings.
To tackle the issue of dealing with unlabeled data,
engineers used techniques to produce pseudo lables which help the
AI model make better predictions. In short, this solution involved
taking the “unknown” proteins, making predictions on them, then
taking the proteins that have been predicted positive and
feeding them into the model again for training, but this time as
labeled examples.
results
Of the 20,000 proteins, each with 20,000 unique features, the AI/ML system the Fuse Team developed in 4 months predicted and ranked an ordered list of the top 100 proteins with rejuvenating properties the clients requested. Out of the 10 proteins that were chosen to conduct experiments on, 8 were found to be true positives, far exceeding the clients expectation of 1.